Thank you for visiting nature.com. You are using a browser version with limited support for CSS. To obtain the best experience, we recommend you use a more up to date browser (or turn off compatibility mode in Internet Explorer). In the meantime, to ensure continued support, we are displaying the site without styles and JavaScript.
Communications Earth & Environment volume 4, Article number: 123 (2023 ) Cite this article Livestock And Animal Husbandry

Animal feces from livestock farming can be a major source of antibiotic resistance to the environment, but a clear gap exists on how the resistance reservoir in feces alters as farming activities intensify. Here, we sampled feces from eight Chinese farms, where yak, sheep, pig, and horse were reared under free-range to intensive conditions, and determined fecal resistance using both genotype and phenotype approaches. Animals reared intensively exhibited increased diversity of antibiotic resistance genes and greater resistance phenotypes in feces, which were cross-correlated. Furthermore, at the metagenome contig level, antibiotic resistance genes were co-located with mobile genetic elements at a higher frequency (27.38%) as farming intensified, with associated resistance phenotypes being less coupled with bacterial phylogeny. Intensified farming also expanded the multidrug resistance preferentially carried on pathogens in fecal microbiomes. Overall, farming intensification can increase antibiotic resistance genotypes and phenotypes in domestic animal feces, with implications for environmental health.
Animal feces are often used for the nutritional enrichment of agricultural soils; however, fecal matter also can be a major reservoir of antibiotic-resistant bacteria and antibiotic resistance genes (ARGs)1. Within the framework of the One Health approach, which recognizes the interconnectedness of human, animal, and environmental health, there is a growing concern for antibotic resistance in farm waste and its dissemination risks2. The abundance of ARGs in feces taken from intense pig-breeding operations can be hundreds to thousands of times greater than background soils3; feces from dairy cattle has been shown to serve as a reservoir for 329 ARGs, conferring resistance to 17 classes of antibiotics4; and consequential detection of tetracycline and aminoglycoside ARGs has been reported in feces from commercial poultry and turkey flocks5. However, domestic animals are often reared and treated under very different farming management systems. Fecal resistance and the potential for ARG transmission based on the point of origination have been largely overlooked6,7.
Compared to free-range management, intensification in animal production operations is increasing, as a direct response to rapidly growing human and animal populations8. Of the predicted global increase in antibiotic use by 2030, it is suggested that 2/3 is projected to be due to increasing numbers of domestic animals, and the remaining 1/3 is likely to occur because of shifts in farming toward more intensive systems9. Intensification will demand greater use of antibiotics to combat infectious diseases10, and incomplete metabolism of these antibiotics means that up to 90% of those used may be excreted through feces11. In light of the United Nations’ recent statement that solutions to increasing antibiotic resistance must begin with prevention12, there are critical considerations that should be taken into account if intensification of animal production operations is pursued.
Microbes in these feces readily share genetic information by capturing ARGs from chromosomes and horizontally transferring them via mobile genetic elements (MGEs)13, allowing an increase in antibiotic resistance levels and resulting in multiple resistance1. These animal feces are widely applied to agricultural lands as fertilizer, frequently leading to enrichment of ARGs in fertilized soil14,15 and in plant root and leaf endophytes15. Moreover, many pathogens in animal feces are of human clinical importance via ingestion of fecal contaminated food16. The ongoing intensification of farming worldwide fuels interest in expanded resistance reservoirs, dissemination risk, and their potential link with MGEs and possible pathogens.
In low-to-middle income countries, intensification of farming is especially accelerating due to a scarcity of land and increasing human populations17. For instance, on the remote Qinghai-Tibet Plateau of China, large populations of livestock are freely reared, some of which have exceeded the rearing capacity of local grasslands18. The relocation of residents to rural‒urban areas and the accompanying transformation of traditional farming into intense types is being greatly encouraged by governments. With a general trend toward intensification in the region, it has become critical to know how this might affect resistance development.
Here, we systematically compared antibiotic resistance genotypes and phenotypes in fecal samples taken from eight typical Chinese farms in the southwestern rim of the Qinghai-Tibet Plateau. The farms differed in degree of intensification (free-range, small-scale, and intense) and the type of animal reared (i.e., yak, sheep, pig, and horse, Supplementary Fig. 1). The resistomes and mobilomes of microbial assemblages and their associations in the feces were assessed in silico. In parallel, the resistance phenotypes of bacterial strains isolated from culture media were assessed in vitro. For both resistance genotypes and phenotypes, the potential for pathogens to serve as ARG carriers was further assessed across different farming practices. Our study suggests the substantially enhanced multidrug resistances from genotype to phenotype in the entire fecal microbiomes as animal farming intensified.
Feces from farms employing intense farming had higher (p < 0.05) levels of micro- and meso-elements (including calcium, magnesium, and sulfur) than lower intensity farms but did not significantly differ (p > 0.05) in macro-element levels (e.g., carbon, Supplementary Fig. 2). Of the heavy metals tested, copper and cadmium were higher (p < 0.001) in animal feces from the farms with intensive farming compared with free-range animals. Furthermore, zinc was exceedingly high in feces from the intense pig farm (10.49 mg g−1 on average, p < 0.001), whereas it was almost undetectable in other fecal samples (Supplementary Fig. 3).
Of the 34 antibiotics examined, an average of 10 were detected in the samples (Supplementary Table 1 and Supplementary Table 2). Feces from pen-reared animals had higher concentrations of most detected antibiotics, although many antibiotics (such as aminoglycosides, sulfonamides, and tetracyclines) were also detected in free-range fecal samples. In this case, oxytetracycline was found as high as 33.92 mg kg−1 in samples from intensively farmed pigs, whereas it averaged 0.119 mg kg−1 in the free-range samples.
Fecal resistomes were profiled using high-throughput qPCR targeting 317 ARGs and 57 MGEs. Of these, 207 ARGs and 42 MGEs were detected in all samples, which conferred resistance to 11 major classes of antibiotics and four classes of mobility genes, respectively (Fig. 1 and Supplementary Data 1). Fecal samples from free-range yaks harbored the lowest number of ARGs (34 on average), whereas the highest number (137) was found in intensely farmed pigs (Fig. 1a). The mean relative abundance of detected ARGs also was highest in intense farm pig samples, 46.0 and 10.1 times higher (p < 0.001) than free-range and small-scale farm samples, respectively (Fig. 1b, except for sheep from small-scale farms). When comparing animal species, pig feces always had the most diverse resistomes, regardless of farming type. When total ARGs were grouped into the 11 major types, those conferring resistance to aminoglycoside, macrolide-lincosamide-streptogramin B (MLSB), multidrug, sulfonamide, and tetracycline particularly increased in relative abundance with intensification of farming (Fig. 1c).
a Number of ARGs detected by antibiotic class. b Mean relative abundance of all detected ARGs. For each detected ARG, the relative abundance is estimated as its copy number normalized to that of the bacterial 16S rRNA gene. c Heatmaps of ARG relative abundance throughout all samples by antibiotic class. d–f Detected gene number, mean relative abundance, and their distributional heatmaps in each classification of MGEs. g Linear correlation between the relative abundance of ARGs and that of MGEs. h A comparison of their relative abundance along the intensification gradient. If not specified otherwise, all error bars shown in figures are the standard error. Pairwise differences among samples are indicated by different letters above the error bars.
As with ARGs, increasing numbers of MGEs were found in samples from farms with intensive farming practices (Fig. 1d, e). For instance, 28 MGEs were detected in samples from intensively farmed pigs, compared with 15 in small-scale farm pig samples (Fig. 1d). Transposase MGEs displayed the largest differences in relative abundances between different farming types (Fig. 1f). The mean relative abundance of ARGs was linearly correlated with MGE abundance (R = 0.826, p < 0.001, Fig. 1g), but increases in the relative abundance of MGEs in intensive farming systems were much greater than associated increases in ARGs (Fig. 1h). The absolute abundances of all genes were further quantified, with similar patterns of ARGs and MGEs with increased intensification (Supplementary Fig. 4).
Multivariate statistical analysis further revealed a clear divergence (p < 0.001) of captive farm animal samples from free-range samples. The degree of intensification explained most of the ARG variance (29.2%), with animal species explaining the second largest amount of variance (23.0%, Supplementary Fig. 5).
The structure of bacterial communities in fecal samples was analyzed using 16S rRNA-based sequencing. A total of 5,147 operational taxonomic units (OTUs) were obtained and exhibited distinct clusters according to farming intensity along the first axis of our nonmetric multidimensional scaling analysis (Fig. 2a, b). All fecal samples were dominated by the phyla Firmicutes and Bacteroidetes and the genus Clostridium, but with variations at the genus level specific to animal type and its origin (Fig. 2c, d).
a A nonmetric multidimensional scaling (NMDS) analysis of bacterial communities at the OTU level using Bray‒Curtis distances. b Axis-1 scores of NMDs for all fecal samples. c, d Bacterial community composition at the phylum and genus levels. “Others” is the sum of other taxa with relative abundances less than 1%. e Variation partitioning analysis for determination of the contributions of different assembled factors to the variation in ARGs. f A random forest algorithm (RFA) was used to link ARGs with the 100 most abundant genera. All detected ARGs were examined using RFA, with ARGs that were accurately predicted using certain genera being visualized using heatmaps. Heatmaps show the importance scores (scaled from 0 to 100) of genera that were selected by RFA as biomarkers (with importance scores >60).
The phenotypes of these OTUs were then predicted using the BugBase algorithm. Of the 8 derived phenotypes, there was a much greater abundance of bacteria carrying MGEs in samples from captive animal samples than in those from free-range samples (p < 0.001; except for small-scale sheep farming), with the highest number of bacteria predicted to carry MGEs in pig feces from intensive farms (Supplementary Fig. 6). Other phenotypes were not as obviously dependent on the degree of farming intensification.
To investigate the drivers of fecal bacterial resistance, variation partition analysis was first used (Fig. 2e). The ARG variation can be exclusively explained by basic and nutritional properties (15.3%), antibiotics (22.8%), and heavy metals (5.64%). When all abiotic factors are considered, 13.6% of the ARG variances remained unexplained; however, when the bacterial community composition was incorporated into the model, it explained an additional 11.3% of the variance, leaving only 2.31% unexplained. This suggests that altered bacterial resistance was best explained by interactions between abiotic factors that exert different selective pressures and variations in bacterial community composition that influence gains or losses in host-dependent resistance.
Based on the above findings, we applied a machine-learning approach to show potential correlations of bacterial taxa with ARGs. Here, two groups of interest were examined: dominant genera (top 100 in relative abundance, Fig. 2f and Supplementary Fig. 7) and pathogens (35 derived, Supplementary Fig. 8). Pathogens were derived here by searching the 16S rRNA-derived OTUs against a bacterial pathogen database. We found that pathogens made up an increasingly high proportion of the total bacterial communities as farming intensified (Supplementary Fig. 8a). Only 14 ARGs, most of which conferred resistance to aminoglycoside and tetracycline, could be accurately predicted from the dominant genera, whereas 27 ARGs could be predicted by the pathogens. Furthermore, a large proportion of ARGs that can be predicted by pathogens belong to the multidrug-associated class. Herein, Escherichia coli, Bacteroides spp., and Clostridium perfringens were identified as possible predictors for resistance. For instance, numerous multidrug resistance genes (e.g., mdtH, arcA, and acrF) can be predicted by Escherichia coli, and Bacteroides spp. often were found to be suggested hosts for many kinds of ARGs.
The abundance of most taxa that served as predictors of ARGs (>85%) was positively associated with ARG abundance (rather than negatively associated), as revealed by partial dependence plots. Moreover, many selected predictors (e.g., Bacteroides spp. and Clostridium perfringens) had increased abundance or became dominant in feces in intensively farmed animals, suggesting these taxa are possible hosts of ARGs (Supplementary Fig. 7 and Supplementary Fig. 8).
Shotgun metagenome sequencing then was performed on fecal samples. Metagenomics confirmed the results from high-throughput qPCR in that feces from intensive farms harbored higher proportions of ARGs and MGEs (Fig. 3a, b and Supplementary Data 2). For example, integrases were almost absent in feces from free-range farms, but they were very prevalent in feces from intensive pig farms. At the contig level, ARGs co-located with MGEs on the same contigs were at a higher frequency (27.38% on average) in samples from captive farms compared with free-range farms (17.52%, Fig. 3c). A total of 38.6% of ARG-containing contigs harbored MGEs in feces from intensively farmed pigs. For these feces, a frequent conjunction type was multiple transposases combined with ARGs conferring resistance to aminoglycoside, sulfonamide, and tetracycline (Fig. 3c). As an example, a dominant contig (e.g., Scaff102014) carried aminoglycoside genes together with the IS6 family transposon and integrase intI on the same contig.
a Mean ARG abundance (TPM, normalized relative reads per kilobase million) and by antibiotic class. b Mean MGE abundance (TPM) by integrase, transposase and insertional, as well as plasmids. c MGEs co-located with ARGs. Proportion of co-occurring MGE plus ARG contigs in all antibiotic resistance contigs (left). Several dominant contigs derived from feces in intense farm pigs are exhibited to show the arrangements of multiple antibiotic resistance open reading frames (ARG-like ORFs) or ARG-like ORFs plus MGE-like ORFs located on the same contig (right). d Potential bacterial hosts harboring ARGs. The percentage distribution of ARG hosts (left). Of all identified hosts, the proportion of potential bacterial pathogens (right).
At the genus level, except for unannotated contigs (54.9%), a majority of ARG-carrying contigs were assigned to Escherichia (6.60%), Clostridium (4.20%), and Bacteroides (3.64%). Furthermore, for those annotated as ARG-carrying species, 81.2% and 69.6% could be classified as possible bacterial pathogens in samples from intensive pig farms and small-scale yak operations, respectively, while the percentage averaged 31.6% in samples from all free-range farms (Fig. 3d).
Resistance phenotypes were examined by performing the standard disc diffusion assay on a total of 1324 bacterial isolates, all belonging to four phyla (Proteobacteria, Firmicutes, Actinobacteria, and Bacteroidetes), to assess relative susceptibility to the 34 antibiotics. These isolates included a high proportion of gram-negative species and a high proportion of opportunistic pathogenic genera in feces from captive animals (Fig. 4a). Mean resistance phenotyping (indicated by mean IZD values per sample) showed higher resistance in species isolated from captive animal samples, whereas background soil samples displayed much lower phenotypic resistance (i.e., the highest IZD values, Fig. 4b). Among the test antibiotics, fecal isolates exhibited the highest resistance to sulfonamides, as well as to several tetracyclines and aminoglycosides (Fig. 4c).
a Phylum-level composition of strains collected from each sample. These strains were then classified as gram-positive (G+), gram-negative (G-), and undetermined (UD). The percentage of opportunistic pathogens among these strains is shown. b The mean diameter of the inhibition zone (IZD) for each sample. The differences between samples were tested as in other figures. c The mean IZD for each antibiotic tested given in increasing order. d Density plot showing the numbers of antibiotic classes to which each isolate is resistant (resistance antibiogram). For the above illustrations, soils collected from agricultural paddy lands in the Yunnan Hani terrace were used as out-tree references. e Occurrence (%) of multidrug-resistant isolates within all isolates. MDR, multidrug-resistant; XDR, extensively drug-resistant; PDR, pan-drug-resistant. f Effect sizes for IZD differences in isolates of opportunistic pathogenic genera Acinetobacter, Bacillus, Enterobacteriaceae, Pseudomonas, and Enterococcus from feces between free-range and captive (small-scale and intense) farms. Circles in red represent significant effect sizes.
The mean number of antibiotic classes to which isolates were typically resistant was 4.0, 5.6, and 6.4 for soil, free-range, and captive animal samples, respectively, and resistance antibiograms showed a clear shift toward higher levels of multiple resistance from control soils to the fecal samples from intensive pig farms (Fig. 4d). Based on the resistance antibiograms, a multi-antibiotic resistance index, the ratio between the number of resistant bacterial isolates and the total number of combinations tested [number of resistant bacterial isolates / (number of antibiotics * number of isolates tested)], was calculated to be 0.30 for soil samples and 0.43, 0.53, and 0.58 for free-range, small-scale, and intensive farm fecal samples, respectively. In addition, most isolates (84.6 to 100%) were found to be multidrug-resistant, although none were pan-drug-resistant (Fig. 4e). Compared to those isolated from soils, some fecal isolates (8.33 to 47.0%) were identified as being extensively drug-resistant, with the highest numbers found in yak samples from small-scale operations.
Resistance phenotypes were then compared individually for isolated dominant taxa at the genus level. Feces from captive animals showed broadly enhanced resistance phenotypes to our test antibiotics, but resistance patterns were highly genus specific. For instance, some nonpathogenic taxa, such as Brevundimonas, Stenotrophomonas, and Sphingomonas, showed greater resistance to multiple antibiotics, whereas other taxa did not (e.g., Lysinibacillus or Arthrobacter, Supplementary Fig. 9). Greater resistance was often found in opportunistic pathogenic genera, such as Acinetobacter, Bacillus, and Enterococcus, which had diverse patterns of resistance. Conversely, other groups (e.g., Pseudomonas and Enterobacteriaceae) did not exhibit significantly greater resistance to most of the antibiotics tested (Fig. 4f). Despite the high predicted prevalence of Escherichia coli as a host of ARGs with metagenomic analyses, this prevalence was not seen in the isolates, which may be due to the low number of strains isolated.
ARG, antibiotic resistance bacteria, and MGE abundances were all greater in animal feces from farms with intensive practices. To understand the ecological potential for multidrug resistance, co-occurrence patterns of ARGs were determined using Chi-square (χ2) and Spearman’s tests. As shown, co-occurring ARG pairs (as indicators of multiple resistance) were much lower in free-range samples than in captive animals (χ2: 779 vs. 3,035 for number of significant pairs; Spearman: 6.770 vs. 33.58 for average connectivity, Supplementary Fig. 10). Since frequent detection of some ARGs can mislead estimations of multidrug resistance, a ratio was introduced in this study to quantify the portion of multiple resistance to antibiotics from different classes as normalized to those from the same antibiotic class (see Methods). The co-occurrence of ARGs between antibiotic classes in captive animal fecal samples was more evident than in free-range samples: 5.620 versus 2.885 using χ2 tests and 5.398 versus 4.133 using Spearman’s tests (Ratiomulti, Supplementary Fig. 10).
Co-occurrence patterns of phenotypic resistance for pairs of antibiotics also were assessed using these two statistics (χ2 and Spearman’s tests, Fig. 5a, b). The number of significant pairs derived from χ2 tests was nearly double in samples from captive animals than in those from the soil control and free-range samples. Co-occurrence of resistance phenotypes was also differentially distributed within and between antibiotic classes, toward a relatively higher co-occurrence of resistance occurring in different antibiotic classes in captive samples.
a Co-occurrence networks based on Spearman’s correlation coefficient r for determining inhibition zone diameter values. The network index (i.e., average connectivity) is calculated. b Co-occurrence heatmaps based on Cramer’s V using χ2 tests for antibiotic-resistant and nonresistant bacteria. The number of significant pairs was calculated. The ratio represents the ratio of “between” to “within” antibiotic classes for the two calculated statistics. c Phylogenetic signal is revealed using Blomberg’s K. For each box, the horizontal bars within boxes represent medians, and the tops and bottoms of the boxes represent the 75th and 25th percentiles, respectively. The upper and lower whiskers extend to 1.5 × the interquartile range. Pairwise differences among samples are indicated by different letters above each box.
Resistance isolates strongly clustered by antibiotic class (i.e., antibiotics within the same class resulted in similar phenotypic patterns, R2 = 0.598–0.649, p < 0.001, Adonis) and, to a lesser extent, clustered according to microbial phylogeny (R2 = 0.006-0.012, p < 0.05, Adonis, Supplementary Fig. 11a, b). The phyla Proteobacteria, Firmicutes, and Actinobacteria all exhibited minor but significantly different patterns of resistance phenotypes between soil and fecal samples from free-range and captive animal samples (Supplementary Fig. 11c), indicating that resistance phenotypes tended to depend on bacterial phylogeny. However, whether intensive farming causes less coupled bacterial phylogeny from resistance because of the prevalence of MGEs is unknown.
To assess this, resistance phenotypes were taken as traits and subjected to phylogenetic signal analyses using Blomberg’s K statistic. The results show that none of the samples had particularly strong phylogenetic signals (<1) of IZD traits; however, among test samples, the highest K values (i.e., the samples with the highest dependence on phylogeny) were observed in soils for most test antibiotics, whereas the lowest were always observed in samples from pen-reared animals and, in a few cases, free-range samples (Fig. 5c).
Most previous studies on fecal samples taken from livestock or poultry farms have used either molecular culture-independent screening or culture-based approaches to evaluate antibiotic resistance in fecal microbiomes. At the farm level, feces from conventional, antibiotic-free, and organic farming have been compared. For example, the most common genes found in feces from commercial poultry flocks from Italy are those against tetracycline and aminoglycosides5. Escherichia coli isolates from antibiotic-free and organic farms exhibit a lower proportion of resistance to antibiotics than isolates from conventional farms, which is of particular concern given the identification of pathogenic Escherichia coli in the latter6. Organic systems also represent a protective barrier to conventional systems due to their low prevalence of resistant Escherichia coli isolates, and high cephalosporin resistance has been detected in conventional samples, despite their prohibition in poultry farming7. Another recent study did not arrive a conclusion that antibiotic-free farming is an effective model for reducing the occurrence of ARGs, but the authors suggest that environmental exposure and the legacy of previous antibiotic use should be considered19. For cattle farming, orders of magnitude lower ARG abundances were detected in waste lagoons from “organic” cattle feedlots compared with feedlots using antibiotics for therapeutic and other purposes20.
While the examples cited above are informative, comparisons of fecal resistance across farming intensification gradients are probably more valuable using combined culture-dependent and -independent approaches, as we have done in this study. Culture-dependent methods inform about specific resistant pathogens of immediate veterinary concern, whereas culture-independent approaches capture a broader range of resistance in organisms that cannot be cultured. Data from both approaches are particularly important in developing countries, which are at the cusp of increasingly intensified farming practice.
Herein, as livestock farming intensified from free-range to intensive systems, higher concentrations of metals and antibiotics were detected in feces. Intensified farming also expanded the resistance reservoir in fecal microbiomes, both genotypically and phenotypically. Upon a close inspection, resistance to three major antibiotic classes (aminoglycosides, tetracyclines, and sulfonamides) was most strongly selected under intensive farming systems. These antibiotics are commonly used in farming and account for more than 50% of the total antibiotic use in animal breeding3,11,21,22. Increased resistance regarding both ARGs and phenotypes mirrored a sharp increase in the concentrations of these antibiotics detected in feces from captive animals compared with free-range animals.
Moreover, ARGs conferring resistance to other antibiotics that were not detected or detected only at low levels across samples (e.g., fluoroquinolones and MLSBs) were also obviously enriched as farming intensified. This implies that some ARGs are probably co-located on the same cassettes and have been co-selected by similar agents. For instance, in an antibiotic-feeding test, aminoglycoside resistance genes were particularly enriched in feces taken from farmed pigs. These genes conferred resistance to aminoglycosides that were not administered on farms in the study23. Similarly, metagenome sequencing revealed that in intensively farmed pig feces, a high co-occurrence of aminoglycosides and other resistance genes (e.g., sulfonamide resistance genes) was apparent on the same contigs.
For the overall map of all detected ARGs, farming intensification explained most of the ARG variations, followed by animal species, confirming that the expansion of resistomes in fecal bacteria is a result of more intensive farming practices. It is worth noting that free-range animals were not antibiotic-free in the surveyed Tibetan regions. Many antibiotics and ARGs were also detected in free-range samples, implying that these animals also receive exposure to antibiotics. Furthermore, free-range livestock may serve as a source of antibiotic resistance for wildlife that inhabit the same areas in anthropized grazing lands24, and vice versa25. The mass movement and transmission pathways of antibiotic resistance at the interface of wildlife and livestock should be further monitored.
Our study further concluded that the enlarged resistance reservoir under farming intensification is closely associated with increased levels of MGEs. Mechanically, selection for resistant strains is driven not only by mutations but also by the acquisition of mobile elements that confer resistance to multiple antibiotics13. Under strong selective pressure, resistance can increase due to widespread horizontal gene transfer, which usually requires MGEs as carriers13,26. For instance, enteric strains such as Escherichia coli have evolved resistance to the most recent generation of beta-lactam antibiotics by acquiring plasmids carrying extended-spectrum β-lactamases and carbapenemases27. The abundance of MGEs can therefore reflect the horizontal transfer potential of ARGs among different bacterial hosts. Fecal samples from intensively farmed animals harbored substantially greater numbers of MGEs (proportionally much greater than ARGs) than those from free-range animals according to the quantitative PCR-based approach. Metagenomes confirmed that MGEs in feces from intense farms tended to associate with ARGs at a higher frequency on the same contigs and further, the frequent conjunction type was transposases with aminoglycoside, tetracycline, and sulfonamide resistance genes. The prevalence of MGEs associated with intensification will probably increase ARG transfer between microbes, resulting in multidrug resistance.
Since a fresh perspective on the topic of antibiotic resistance stems from the evolutionary comprehension of the emergence of resistant isolates13,28, here we are also interested in investigating the microbial phylogenetic dependence of phenotypic resistances as farming intensified. For soil samples, soil microbiota resistomes are strongly correlated with phylogenetic signatures and ecological divisions28, despite horizontal gene transfer of ARGs. This up-to-date finding suggests that resistance in soils has followed an evolutionary trajectory and is rooted in bacterial phylogeny.
Here in fecal samples, increasing selective pressure with progressive farming intensification appears to consequentially accelerate natural evolutionary processes and decouples fecal resistance from phylogeny to a significant extent. Genes can be mobilized and then penetrate into taxonomically distant bacterial populations13, weakening their previous phylogenetic signatures. This provides further evidence that horizontal gene transfer plays a crucial role in shaping antibiotic resistance in isolated fecal bacteria.
Intensified livestock farming also expands the carriage of multidrug resistance on pathogens in animal feces. Enrichment of lineages of Proteobacteria (especially opportunistic pathogens) in soils has increased the detection of shared resistance between soil and hospital settings29, suggesting that certain opportunistic pathogens in environments can be a conduit through which ARGs are transferred from natural environments to clinics. These environmental isolates, particularly (opportunistic) pathogens, thus have significant clinical relevance30. The combination of ARGs and pathogens in feces will probably lead to greater human health risks due to enrichment and spread.
We explored this combination by showing that intensification can expand pathogen-carried antibiotic resistance. Contigs carrying ARGs were also more consistently assigned to possible pathogenic bacteria in captive versus free-range animals. These ARG-containing contigs were mostly annotated as Escherichia, Clostridium, and Bacteroides spp., which appear to serve as hosts for ARGs, and subsequently risks of resistance transmission are aggravated. These potential ARG hosts were also successfully predicted by using a machine-learning approach from this study. A previous study compared genomes from 433 common human pathogens and 153 nonpathogenic soil microbes, and suggested that ARGs acquired by nonpathogenic soil bacteria (and under low selective pressure) have less potential for gene transfer than those in human pathogens, which are under higher selective pressure28. The presence of pathogens is often found strongly associated with the biopollutant contamination of some ARGs, for example in wastewater treatment systems31. In accordance, here we showed that the potential pathogens, rather than dominant taxa, may be key carriers in the spread of resistance from fecal microbiomes by acting as ARG hosts under intensive farming.
Interestingly, we found that many isolates from captive animal fecal samples (especially from intensive farms) grew slowly and had low viability, and subsequently, fewer strains were isolated compared with those from free-range animals (Fig. 4a). This implies that gaining resistance may cause a fitness cost13, which comes at the expense of viability, although more data are needed to confirm this speculation. Despite potentially reduced fitness, potential pathogenic bacteria in animal feces from the intense farms evolved resistance to diverse antibiotics.
When farming intensified, a concordance in changes between antibiotic resistance genotype and phenotype was observed, specifically for aminoglycoside, sulfonamide, and tetracycline resistance. The detection of higher concentrations of these antibiotics in feces as farming intensified provides evidence that antibiotic pressure contributes to the development of resistance. This resistance development is characterized by higher levels of MGEs, which were typically co-located with ARGs that confer resistance to these antibiotics. At this point, all these data are linked together to show lines of evidence for increased fecal resistance from genotype to phenotype. This study provides the comprehensive report on fecal resistance, both genotypically and phenotypically, from the perspective of the entire fecal microbiomes. It serves as an important complement to the characterization of antibiotic resistance profiles in certain isolates from clinically relevant food-producing animals, such as Escherichia coli32, Staphylococci spp.33, and Salmonella enterica34.
New policy and practice are needed on how to preserve traditional free-range farming and promote the development of improved strategies for the treatment and disposal of animal wastes, particularly from intensive farms35. This is strongly supported by recent UN guidance12 aimed at preventing resistance and promoting One Health-based solutions. In summary, our study suggests substantially enhanced multidrug resistances in fecal microbiomes as livestock farming intensified. This has implications for the transmission and spread of resistance in the future, given that global intensification may become critical to satisfying the world’s food needs.
Animals from eight “typical” farms located in the southwest rim of the Qinghai-Tibet Plateau in Southwest China, were surveyed (Supplementary Fig. 1). Four farms are alpine meadow pastures, where local farmers graze “free-range” yaks, sheep, pigs, and horses. Such farming is representative of current Qinghai-Tibet Plateau practices. An additional three farms were assessed that were managed by dealers or families for the “small-scale” rearing of yak, sheep, and pigs (<500 animals per farm) in villages, which is common in rural Chinese areas. The eighth farm is a typical “intense” pig-rearing operation (>10,000 animals) with modern close-end management. The small-scale and intense farms both employed captive farming practices and had greater herd densities.
Fresh feces shed by six healthy, mature animals per farm (n = 6) were collected in May 2020, providing 48 fecal samples from selected animals from different farming types. Samples were kept on dry ice for transport to the lab and were subsequently split and either stored at −80 °C for physicochemical analyses and DNA extraction or at 4 °C for immediate isolation of bacterial strains. In the assay of resistance phenotype profiling, a pooled soil sample (comprised of 10 blended subsamples) taken from paddy lands in the Hani Terrace (Yunnan Province, China) was also examined and compared with fecal samples to assess differences in resistance phenotypes. Incorporating the soil sample provides another baseline for phenotype comparison.
Fecal basic properties (e.g., pH and organic matter) were quantified following Bao36. Five heavy metals (copper, zinc, lead, cadmium, and chromium) were quantified following previously described methods37. A total of 34 antibiotics within 10 classes (5 aminoglycosides, 8 beta-lactams, 2 fluoroquinolones, 1 glycopeptide, 5 MLSB, 1 polypeptide, 1 phenicol, 5 sulfonamides, 5 tetracyclines, and 1 trimethoprim, Supplementary Table 1) were quantified using an HPLC-MS/MS system (LCMS-8040 system, Shimadzu, Japan) with an ODS column (1.6 μm, 75 × 2 mm) (Shim-pack XR-ODS III). The detailed sample preparation and the optimized gradient elution programs for quantification followed Zhi11,38. Beta-lactams, given their unstable character, were quantified immediately after sample preparation; others were also quantified within 72 h.
Total DNA was extracted from fecal samples using a PowerFecal DNA isolation Kit (MoBio Laboratories, USA) and further purified using a DNeasy PowerClean Pro CleanUp Kit (Qiagen, Germany) once the extracts had visible color. DNA concentrations were determined using a Nanodrop instrument (ThermoFisher, USA).
High-throughput qPCR technology was used to quantify genes of interest from DNA samples, including 317 ARGs that confer resistance to ten major classes of antibiotics, 57 MGEs (genes responsible for horizontal gene transfer between bacteria), and the 16S rRNA gene, on a SmartChip Real-time PCR platform (Takara, Shiga, Japan). Validation and assessment of 375 primer sets was conducted by Looft23 and the PCR conditions followed39. Raw data (Supplementary Data 1, Ct value) were subjected to the delta-delta Ct method to evaluate relative abundance (%) through normalization of the copy numbers of each target gene (indicated by Ct value) to those of bacterial 16S rRNA genes39. The detection limit Ct (31) was taken as a replacement for the genes with no amplification. Each sample was run with three technical replicates, and only where all three showed amplifications was this sample considered to be positive.
To calculate the absolute abundance of each target gene, absolute 16S rRNA gene copy numbers were first determined using a gradient dilution approach on a Bio-Rad CFX96 system (Bio-Rad, Munich, Germany) as previously described40. The relative abundances of ARGs and MGEs were then transformed to absolute copy numbers (copies/g dry soil) by further normalizing to absolute 16 S rRNA gene copy numbers.
Amplicon libraries targeting the V3–V4 region of the bacterial 16S rRNA gene were prepared using the primers 341F (5′-ACTCCTACGGGAGGCAGCAG-3′) and 805R (5′-GGACTACHVGGGTWTCTAAT-3′) for the 48 fecal DNA samples. The libraries were sequenced using 300-bp paired-end sequencing on a MiSeq platform at Majorbio (Shanghai, China); the raw data were deposited in the GenBank SRA database (Bioproject accession no. PRJNA831154).
A total of 2,548,181 paired-read reads from 16S rRNA gene amplicons (an average of 53,087 reads per sample) were obtained. These reads were merged and clustered into OTUs with a 97% sequence identify cutoff using UCLUST41, and the RDP classifier was then used to annotate each representative OTU. Bacterial community compositions were estimated from OTU tables after rarefying the read numbers to the lowest number per sample. To identify potential pathogenic species, OTUs from each sample were searched (E-value <1 × 10−10, sequence identity >99%) against a sequence database containing 538 pathogenic bacterial species42. To infer the phenotypes of these OTUs, the 16S rRNA gene sequences were also run with the BugBase algorithm43. The above analyses were conducted using the QIIME 2.0 pipeline44.
Of the 48 fecal DNA samples, 24 samples (three per farm) were randomly selected for shotgun metagenome sequencing. Shotgun libraries were prepared using an Illumina NEB Next ® UltraTM DNA Library Prep Kit (New England Biomlabs, MA, USA). The libraries were then sequenced with 151-bp paired-end sequencing on an Illumina NovaSeq 6000 platform at Magigen (Guangdong, China). The raw data were deposited in the SRA database under no. PRJNA859407.
After quality control using Trimmomatic (v0.36), an average of approximately 7.14 Gb clean reads per DNA sample were obtained (Supplementary Table 3). Reads were then de novo assembled with both single and mixed strategies using MEGAHIT (v1.0.6). Assembled contigs >500 bp in length were kept, and the open reading frames (ORFs) were then predicted from these contigs using MetaGeneMark (v3.38). Before subsequent annotation, the reference ARG database was downloaded from the Comprehensive Antibiotic Resistance Database (CARD, https://card.mcmaster.ca), and the reference MGE database (constituting the NCBI RefSeq plasmids database, the Isfinder database, and the INTEGRALL database) was downloaded and customized. The protein sequences of the predicted ORFs were annotated based on these databases using BLASTx as implemented in DIAMOND (v0.9.19), where the ARGs or MGEs were identified using a cutoff of 80% identity and an amino acid length larger than 2526. The relative abundances of ARGs and MGEs were then calculated in TPM units (normalized relative reads per kilobase million, Supplementary Data 2), after mapping clean reads to each ARG-like or MGE-like sequence.
Thereafter, each assembled contig that contained ARG-like sequences was searched against the reference MGE database to screen contigs in which ARGs and MGEs were potentially co-located45. Predicted ARG hosts were subsequently obtained by searching ARG-containing contigs against NCBI NR databases; once identified at the species level, the hosts were further searched against the database containing 538 pathogenic bacterial species (shown above) to identify ARG-carrying pathogens of clinical importance in fecal samples.
For the isolation of cultivable bacteria, the suspension from 5 g of homogenized soil samples or animal feces in 45 mL of 0.96% saline was prepared using the standard 10-fold dilution method from 10-1 to 10-8. The Nutrient Agar medium was then dispensed in Petri dishes, a sterile cotton swab was dipped into each diluted suspension, and the inoculum was spread evenly over the entire surface of the agar plate. Morphologically different colonies were picked each day during incubation for 7 days at 30 °C to obtain as many phylogenetically different strains as possible. The purity of each single isolate was examined using the streak plant method, after which the pure isolates were inoculated on the slanted surface of Luria-Bertani (LB) agar medium in test tubes at 4 °C before use46,47.
Both background soil samples and animal feces were used as sources for bacterial isolation, the genomic DNA of each isolate was extracted, and 16S rRNA genes were amplified according to the method described by Feng et al.47. The primers targeting the full-length 16S rRNA genes (27f: 5′-CAGAGTTTGATCCTGGCT-3′; 1942r: 5′-AGGAGGTGATCCAGCCGCA-3′) were used here. After amplification, the 16S rRNA gene was sequenced and then searched against the EzBioCloud database (https://help.ezbiocloud.net) for isolate identification. We initially conducted half-length (single-end) 16S rRNA gene sequencing; full-length (paired-end) sequencing was performed for those with a low matching rate.
A total of 1324 identified isolates (“Top-Hit”, EzBioCloud database) were obtained, and their sequenced reads are provided in Supplementary Data 3. The susceptibility of these strains to the 34 antibiotics was tested using the standard disc diffusion assay to measure the diameter of the inhibition zone (IZD) (Supplementary Data 4). Where any colonies were visible growing within the zone, we considered that there was no zone of inhibition (IZD = 0). Two strains, Escherichia coli (ATCC 25922) and Pseudomonas aeruginosa (ATCC 27853), were used as quality control strains. The mass of antibiotics used on each disc was according to the CLSI guidelines 2011 (http://www.microrao.com). The basic methodology, including the preparation of media and inoculum, application of disks, and inoculation of Mueller-Hinton agar plates, followed Matuschek et al.46.
To assess factors that shaped the fecal resistomes, we performed variation partitioning analysis (VPA) using the package “vegan” in R 3.6.2. A machine-learning approach (random forest algorithm, using the R package “randomForest”) then was used to predict the bacterial hosts of ARGs31. Multidrug resistance was depicted by analyzing the co-occurrence of pairwise ARGs or resistance phenotypes using both χ2 and Spearman’s rank correlation tests using the R package “vcd”. The following equation was constructed here and then used to assess how multidrug resistance varied across the tested antibiotics (Eq. 1):
where X represents any index as a proxy for multidrug resistance (such as the number of significant pairs or average connectivity), calculated for pairwise ARGs or resistance phenotypes conferring resistance to two antibiotics belonging to different antibiotic classes (“Xcalculated between antibiotic classes”)48. We also calculated those conferring resistance to two antibiotics belonging to the same antibiotic class (“Xcalculated within each antibiotic class”) and introduced Ratiomulti, which represents the portion of multidrug resistance against antibiotics of different classes as normalized to that selected for those from the same antibiotic class. Here, a total of 34 test antibiotics from 10 classes were investigated.
Furthermore, the resistance phenotype traits were characterized by producing resistance antibiograms. Dominant isolates, including opportunistic pathogens, then were compared for IZD values between free-range and captive farms. To further investigate the microbial phylogenetic dependence of these phenotypic resistances, we quantified their phylogenetic signal strength using Blomberg’s K in the R package “picante”. The illustrations generated in this study were made using R, Excel, and Graph Prim. Unless otherwise specified, comparisons among samples were performed with the Kruskal–Wallis test followed by the post hoc pairwise test (Method = “wilcox.test). A full description of the statistical analysis can be found in the supplementary files (see Supplementary Methods).
The datasets of Ct values from high-throughput qPCR analyses are shown in supplementary Data 1. The datasets of TPM values from metagenomic sequencing analyses are shown in Supplementary Data 2. All isolated and then identified strains with their sequenced reads are listed in Supplementary Data 3. Supplementary Data 4 contains all recorded diameter of the inhibition zone (IZD) for these strains tested using the standard disc diffusion assay. Supplementary Table 2 shows the concentrations of 34 antibiotics (μg kg-1 dry weight) determined in fecal samples. Supplementary Table 3 shows the basic information of the metagenomic datasets obtained by Illumina shotgun sequencing for 24 fecal samples. All these mentioned datasets are available through Dryad (https://doi.org/10.5061/dryad.p5hqbzktd)49. The raw sequence 16S rRNA have been deposited in the sequence read archive (SRA) database in NCBI under Bioproject accession no. PRJNA831154. The raw sequence data of metagenomes are available under no. PRJNA859407.
Udikovic-Kolic, N., Wichmann, F., Broderick, N. A. & Handelsman, J. Bloom of resident antibiotic-resistant bacteria in soil following manure fertilization. Proc. Natl Acad. Sci. USA 111, 15202–15207 (2014).
Aslam, B. et al. Antibiotic resistance: one health one world outlook. Front Cell Infect Microbiol 11, 771510 (2021).
Zhu, Y. G. et al. Diverse and abundant antibiotic resistance genes in Chinese swine farms. Proc. Natl Acad. Sci. 110, 3435–3440 (2013).
Liu, J. et al. The fecal resistome of dairy cattle is associated with diet during nursing. Nat. Commun. 10, 4406 (2019).
Di Francesco, C. E. et al. Research Note: Detection of antibiotic-resistance genes in commercial poultry and turkey flocks from Italy. Poult Sci 100, 101084 (2021).
Tofani, S. et al. Assessing the load, virulence and antibiotic-resistant traits of ESBL/Ampc E. coli from broilers raised on conventional, antibiotic-free, and organic farms. Antibiotics (Basel) 11, (2022).
Musa, L. et al. Susceptibility of commensal E. coli isolated from conventional, antibiotic-free, and organic meat chickens on farms and at slaughter toward antimicrobials with public health relevance. Antibiotics (Basel) 10, (2021).
Cassman, K. G. & Grassini, P. A global perspective on sustainable intensification research. Nat. Sustainability 3, 262–268 (2020).
Boeckel, T. P. V. et al. Global trends in antimicrobial use in food animals. Proc. Natl Acad. Sci. 112, 5649–5654 (2015).
Maron, D. F., Smith, T. J. S. & Nachman, K. E. Restrictions on antimicrobial use in food animal production: an international regulatory and economic survey. Globalization Health 9, 48 (2013).
Zhi, S. L., Shen, S. Z., Zhou, J., Ding, G. Y. & Zhang, K. Q. Systematic analysis of occurrence, density and ecological risks of 45 veterinary antibiotics: Focused on family livestock farms in Erhai Lake basin, Yunnan, China. Environ. Pollut. 267, 115539 (2020).
Programme UNE. Bracing for superbugs: strengthening environmental action in the One Health response to antimicrobial resistance. Geneva, https://www.unep.org/resources/superbugs/environmental-action (2023).
MacLean, R. C. & Millan, A. S. The evolution of antibiotic resistance. Science 365, 1082–1083 (2019).
Laconi, A. et al. Microbial community composition and antimicrobial resistance in agricultural soils fertilized with livestock manure from conventional farming in Northern Italy. Sci. Total Environ. 760, 143404 (2021).
Zhang, H. et al. Animal manure fertilization promotes antibiotic resistance gene dissemination among manure, soil, and vegetables. Huan Jing Ke Xue 42, 2080–2088 (2021).
Algammal, A. M. et al. Emerging MDR-Mycobacterium avium subsp. avium in house-reared domestic birds as the first report in Egypt. BMC Microbiol. 21, 237 (2021).
Pehrsson, E. C. et al. Interconnected microbiomes and resistomes in low-income human habitats. Nature 533, 212–216 (2016).
Yu, C. Q. et al. Ecological and environmental issues faced by a developing Tibet. Environ. Sci. Technol. 46, 1979–1980 (2012).
Farooq, M. et al. Antibiotic resistance genes occurrence in conventional and antibiotic-free poultry farming, Italy. Animals (Basel) 12, (2022).
Peak, N. et al. Abundance of six tetracycline resistance genes in wastewater lagoons at cattle feedlots with different antibiotic use strategies. Environ. Microbiol. 9, 143–151 (2007).
Kemper, N. Veterinary antibiotics in the aquatic and terrestrial environment. Ecol. Indicators 8, 1–13 (2008).
Pan, X., Qiang, Z. M., Ben, W. W. & Chen, M. X. Residual veterinary antibiotics in swine manure from concentrated animal feeding operations in Shandong Province, China. Chemosphere 84, 695–700 (2011).
Looft, T. et al. In-feed antibiotic effects on the swine intestinal microbiome. Proc. Natl Acad. Sci. 109, 1691–1696 (2012).
Smoglica, C. et al. Microbial community and antimicrobial resistance in fecal samples from wild and domestic ruminants in Maiella National Park, Italy. One Health 15, 100403 (2022).
Lee, S. et al. Transmission of antibiotic resistance at the wildlife-livestock interface. Commun. Biol. 5, 585 (2022).
Lee, K. et al. Mobile resistome of human gut and pathogen drives anthropogenic bloom of antibiotic resistance. Microbiome 8, 2 (2020).
Partridge, S. R., Kwong, S. M., Firth, N. & Jensen, S. O. Mobile genetic elements associated with antimicrobial resistance. Clin. Microbiol. Rev. 31, e00088–00017 (2018).
Forsberg, K. J. et al. Bacterial phylogeny structures soil resistomes across habitats. Nature 509, 612–616 (2014).
Forsberg, K. J. et al. The shared antibiotic resistome of soil bacteria and human pathogens. Science 337, 1107–1111 (2012).
D’Costa, V. M., McGrann, K. M., Hughes, D. W. & Wright, G. D. Sampling the antibiotic resistome. Science 311, 374–377 (2006).
Sun, Y. P., Clarke, B., Clarke, J. & Li, X. Predicting antibiotic resistance gene abundance in activated sludge using shotgun metagenomics and machine learning. Water Res. 202, 117384 (2021).
Guerra, B. et al. Phenotypic and genotypic characterization of antimicrobial resistance in German Escherichia coli isolates from cattle, swine and poultry. J. Antimicrob. Chemother. 52, 489–492 (2003).
Wuytack, A. et al. Non-aureus staphylococci in fecal samples of dairy cows: First report and phenotypic and genotypic characterization. J. Dairy Sci. 102, 9345–9359 (2019).
Molla, B. et al. Salmonella enterica in commercial swine feed and subsequent isolation of phenotypically and genotypically related strains from fecal samples. Appl. Environ. Microbiol. 76, 7188–7193 (2010).
Ma, Z., Lee, S. & Jeong, K. C. Mitigating antibiotic resistance at the livestock-environment interface: a review. J. Microbiol. Biotechnol. 29, 1683–1692 (2019).
Bao, S. D. Agro-chemical Analysis of Soil (China Agricultural Press, 2000).
Li, B., Wang, H., Yu, Q. G., Wei, F. & Zhang, Q. Ecological assessment of heavy metals in sediments from Jianhu Lake in Yunnan province, China. Polish J. Environ. Stud. 29, 4139–4150 (2020).
Zhi, S. L., Zhou, J., Yang, F. X., Tian, L. & Zhang, K. Q. Systematic analysis of occurrence and variation tendency about 58 typical veterinary antibiotics during animal wastewater disposal processes in Tianjin, China. Ecotoxicol. Environ. Safety 165, 376–385 (2018).
Chen, Q. L. et al. Long-term field application of sewage sludge increases the abundance of antibiotic resistance genes in soil. Environ. Int. 92-93, 1–10 (2016).
Wang, H., Qi, J. F., Xiao, D. R., Wang, Z. B. & Tian, K. A re-evaluation of dilution for eliminating PCR inhibition in soil DNA samples. Soil Biol. Biochem. 106, 109–118 (2017).
Edgar, R. C. Search and clustering orders of magnitude faster than BLAST. Bioinformatics 26, 2460–2461 (2010).
Woolhouse, M. E. J. & Gowtage-Sequeria, S. Host range and emerging and reemerging pathogens. Emerg. Infect. Dis. 11, 1842–1847 (2005).
Ward, T. et al. BugBase predicts organism-level microbiome phenotypes. bioRxiv https://doi.org/10.1101/133462 (2017).
Caporaso, J. G. et al. QIIME allows analysis of high-throughput community sequencing data. Nature Methods 7, 335–336 (2010).
Liang, J. S. et al. Identification and quantification of bacterial genomes carrying antibiotic resistance genes and virulence factor genes for aquatic microbiological risk assessment. Water Res. 168, 115160 (2020).
Matuschek, E., Brown, D. F. J. & Kahlmeter, G. Development of the EUCAST disk diffusion antimicrobial susceptibility testing method and its implementation in routine microbiology laboratories. Clin. Microbiol. Infect. 20, O255–O266 (2014).
Li, L. L. et al. Propioniciclava soli sp. nov., isolated from forest soil, Yunnan, China, and reclassification of the genus Brevilactibacter into the genus Propioniciclava, and Brevilactibacter sinopodophylli, Brevilactibacter flavus, and Brevilactibacter coleopterorum as Propioniciclava sinopodophylli comb. nov., Propioniciclava flava comb. nov., and Propioniciclava coleopterorum comb. nov., respectively. Arch. Microbiol. 204, 39 (2021).
Tanwar, J., Das, S., Fatima, Z. & Hameed, S. Multidrug resistance: an emerging crisis. Interdiscip. Perspect. Infect. Dis. 2014, 541340 (2014).
Wang, H. et al. (2023), Intensified livestock farming increases antibiotic resistance genotypes and phenotypes in animal feces. Dryad, Dataset, https://doi.org/10.5061/dryad.p5hqbzktd (2023).
This work was supported by the Urban/peri-urban soil biogeochemical processes and environmental quality (4202100004), National Natural Science Foundation of China (32160311 and 41877346) and China Postdoctoral Science Foundation (2020M671952). We thank Ba Ruo for his help with the sampling, Juan Yang for her assistance with antibiotic analyses, Ming-Gang Li and Pei-Wen Yang for their guidance in bacterial strain culture and isolation, and Wenshan Li and Wanzhe Cui for the identification of isolates and construction of bacterial phylogenetic trees.
These authors contributed equally: Hang Wang, Jin-Feng Qi.
Key Lab of Urban Environment and Health, Institute of Urban Environment, Chinese Academy of Sciences, 361021, Xiamen, People’s Republic of China
Hang Wang, Kai Ding & Yong-Guan Zhu
Yunnan Key Laboratory of Plateau Wetland Conservation, Restoration and Ecological Services, National Plateau Wetlands Research Center, Southwest Forestry University, 650224, Kunming, People’s Republic of China
Hang Wang & Rong Qin
Department of Economic Plants and Biotechnology, Yunnan Key Laboratory for Wild Plant Resources, Kunming Institute of Botany, Chinese Academy of Sciences, 650201, Kunming, People’s Republic of China
School of Engineering, Newcastle University, Newcastle upon Tyne, NE1 7RU, UK
You can also search for this author in PubMed Google Scholar
You can also search for this author in PubMed Google Scholar
You can also search for this author in PubMed Google Scholar
You can also search for this author in PubMed Google Scholar
You can also search for this author in PubMed Google Scholar
You can also search for this author in PubMed Google Scholar
Y.G.Z. designed the project. H.W. and R.Q. collected and processed the fecal samples. H.W. performed high-throughput qPCR measurements and DNA sequencing. J.F.Q. conducted HPLC‒MS/MS and bacterial strain isolation and identification analyses. H.W. and J.F.Q. analyzed the data, prepared the figures, and generated the first draft of the manuscript. D.W.G., Y.G.Z., and K. D. contributed by commenting on and revising it. All authors read and approved the final manuscript.
The authors declare no competing interests.
Communications Earth & Environment thanks the anonymous reviewers for their contribution to the peer review of this work. Primary Handling Editor: Aliénor Lavergne. Peer reviewer reports are available.
Publisher’s note Springer Nature remains neutral with regard to jurisdictional claims in published maps and institutional affiliations.
Open Access This article is licensed under a Creative Commons Attribution 4.0 International License, which permits use, sharing, adaptation, distribution and reproduction in any medium or format, as long as you give appropriate credit to the original author(s) and the source, provide a link to the Creative Commons license, and indicate if changes were made. The images or other third party material in this article are included in the article’s Creative Commons license, unless indicated otherwise in a credit line to the material. If material is not included in the article’s Creative Commons license and your intended use is not permitted by statutory regulation or exceeds the permitted use, you will need to obtain permission directly from the copyright holder. To view a copy of this license, visit http://creativecommons.org/licenses/by/4.0/.
Wang, H., Qi, JF., Qin, R. et al. Intensified livestock farming increases antibiotic resistance genotypes and phenotypes in animal feces. Commun Earth Environ 4, 123 (2023). https://doi.org/10.1038/s43247-023-00790-w
DOI: https://doi.org/10.1038/s43247-023-00790-w
Anyone you share the following link with will be able to read this content:
Sorry, a shareable link is not currently available for this article.
Provided by the Springer Nature SharedIt content-sharing initiative
By submitting a comment you agree to abide by our Terms and Community Guidelines. If you find something abusive or that does not comply with our terms or guidelines please flag it as inappropriate.
The real story behind the research, from conception to publication, the highs and the lows
Communications Earth & Environment (Commun Earth Environ) ISSN 2662-4435 (online)
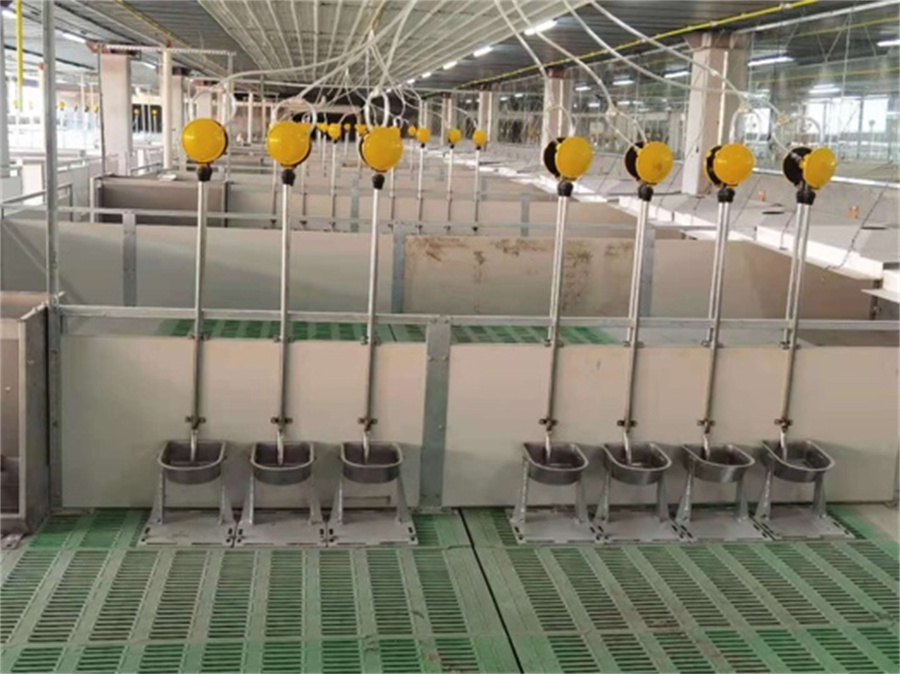
Elevated Farrowing Pen Sign up for the Nature Briefing newsletter — what matters in science, free to your inbox daily.